Overview
In this workshop we will discuss the importance of uncertainty in deep learning for robotic applications. Invited expert speakers will discuss the importance of uncertainty in deep learning for robotic perception, but also action. In addition the workshop will provide a forum to discuss novel and ongoing work.
In addition, the workshop will introduce two new research challenges and competitions:
- The Probabilistic Object Detection challenge, a new challenge that evaluates the ability of visual object detectors to accurately quantify their spatial and semantic uncertainty.
- The Robotic Vision Scene Understanding Challenge evaluates how well a robotic vision system can understand the semantic and geometric aspects of its environment.
Participate
The workshop will take place in Room L1-R6, on 8 November 2019.
Post your questions for the panel discussion on slido, using the event code #IROS-Uncertainty.
Schedule
Our workshop features talks by four invited speakers in the morning, followed by a panel discussion before we break for lunch. In the afternoon, the authors of the contributed papers present their work in talks and an interactive poster session.
Please join us 8 November in Room L1-R6.
Time | Event |
---|---|
09:00 | Welcome, Introduction, Overview |
09.15 | Hermann Blum (ETH Zürich): How well does uncertainty estimation actually work? |
09:45 | Fabio Ramos (NVIDIA, University of Sydney): Inferring the uncertainty of simulator parameters for Sim2Real and deep RL |
10:15 | Di Feng (Bosch): Towards Safe Autonomous Driving: Capture Uncertainty in Deep Object Detectors |
10:45 | Coffee Break |
11:15 | Krzysztof Czarnecki (University of Waterloo): Uncertainty-Centric Safety Assurance of ML-Based Perception for Automated Driving |
11:45 | Workshop Organisers: Probabilistic Object Detection and Scene Understanding: Two new Research Challenges and Competitions. |
12:15 | Panel Discussion. Use event code #IROS-Uncertainty to post your questions on slido. |
12:45 | Lunch Break |
14:00 | Youngji Kim, Sungho Yoon, Sujung Kim, Ayoung Kim (KAIST and Naver Labs): Balanced Covariance Estimation for Visual Odometry Using Deep Networks. |
14:15 | Ali Harakeh, Steven L Waslander. (University of Toronto): How Should We Evaluate Probabilistic Object Detectors? |
14:30 | Junjiao Tian, Wesley Cheung, Nathan Glaser, Yen-Cheng Liu, Zsolt Kira (Georgia Institute of Technology): UNO: Uncertainty-aware Noisy-Or Multimodal Fusion for Unanticipated Input Degradation. |
14:45 | Andrea De Maio, Simon Lacroix (LAAS-CNRS): On learning visual odometry errors. |
15:00 | Closing Remarks |
15:10 | Poster session |
Organisers
The Robotic Vision Challenges organisers are with the Australian Centre for Robotic Vision at Queensland University of Technology (QUT), Monash University, the University of Adelaide, and Google AI.
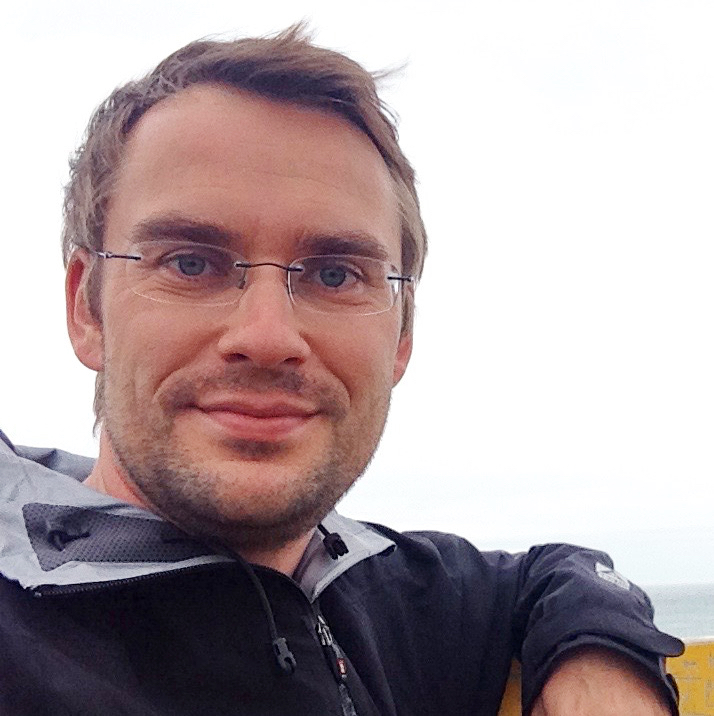
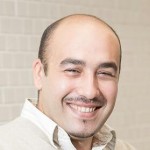
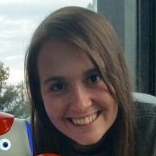
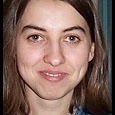
Queensland University of Technology
Queensland University of Technology
Queensland University of Technology
Google Brain
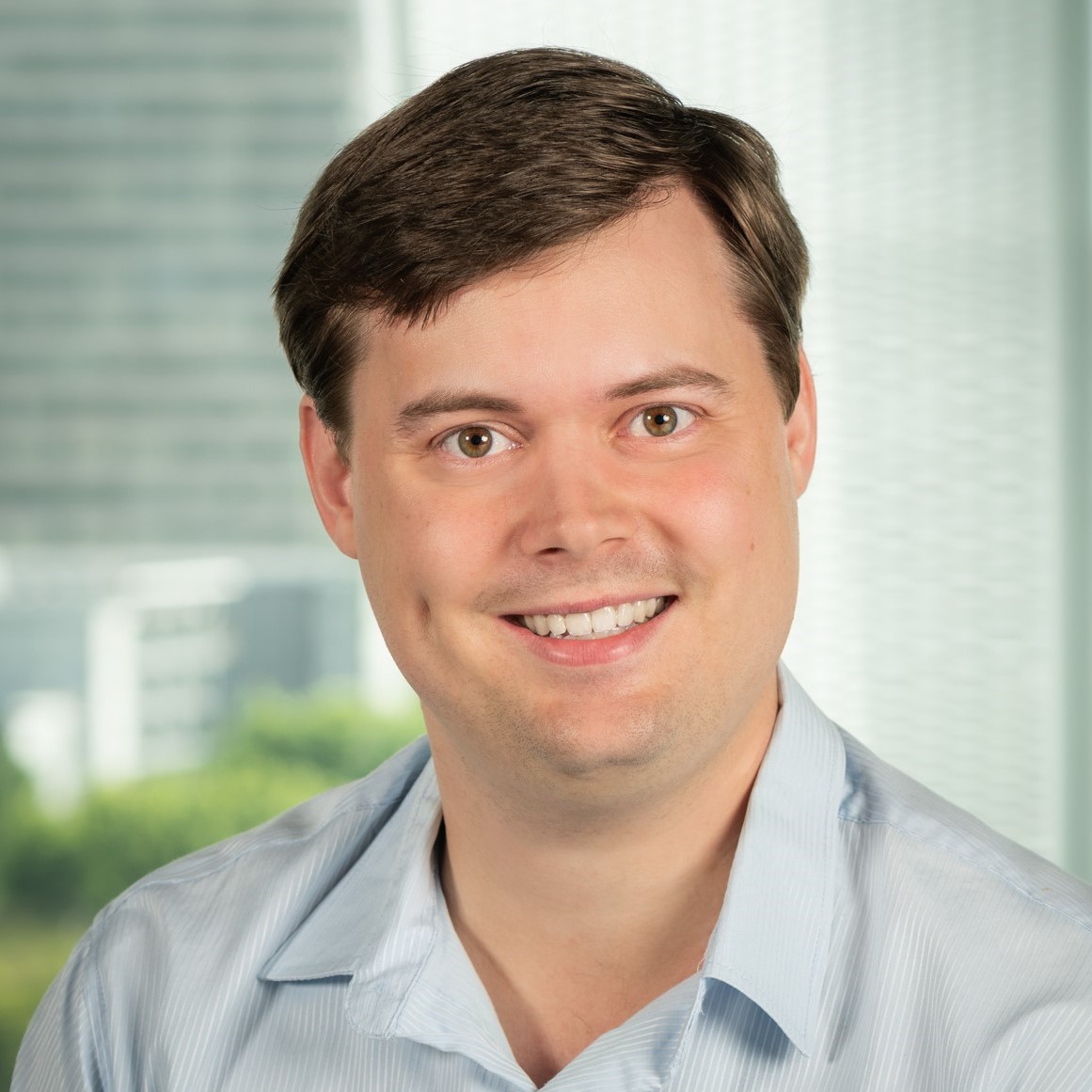
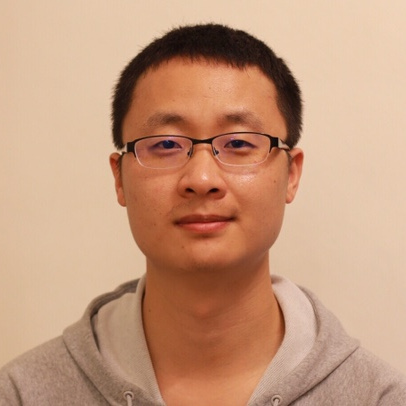
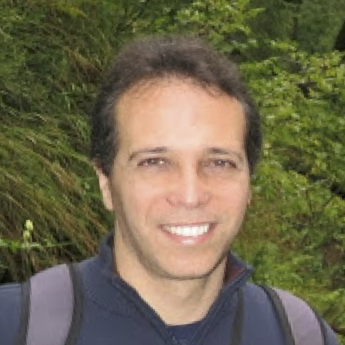
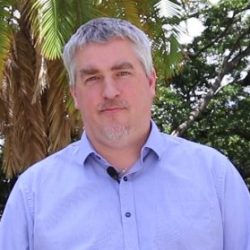
Queensland University of Technology
Queensland University of Technology
University of Adelaide
Monash University