Introduction
This workshop will bring together the participants of the first Robotic Vision Challenge, a new competition targeting both the computer vision and robotics communities.
The new challenge focuses on probabilistic object detection. The novelty is the probabilistic aspect for detection: A new metric evaluates both the spatial and semantic uncertainty of the object detector and segmentation system. Providing reliable uncertainty information is essential for robotics applications where actions triggered by erroneous but high-confidence perception can lead to catastrophic results.
Program: 17 June 2019, Room 103B
Our workshop will concentrate on the outcomes of the first Probabilistic Object Detection Challenge in the morning session, featuring talks by the four best scoring teams. After that we welcome our invited speakers.
- 09:00 - 09:30 - Welcome, Introduction, Overview, Results Summary
- 09:30 - 09:45 - Contributed Talk (1st place): AugPOD: Augmentation-oriented Probabilistic Object Detection. Chuan-Wei Wang, Chin-An Cheng, Ching-Ju Cheng, Hou-Ning Hu, Hung-Kuo Chu, Min Sun.
- 09:45 - 10:00 - Contributed Talk (2nd place): A Mask-RCNN Baseline for Probabilistic Object Detection. Phil Ammirato, Alexander C. Berg.
- 10:00 - 10:45 - Coffee Break
- 10:45 - 11:00 - Contributed Talk (3rd place): Probabilistic Object Detection via Staged Non-Suppression Ensembling. Dongxu Li, Chenchen Xu, Yang Liu, Zhenyue Qin.
- 11:15 - 11:30 - Contributed Talk (4th place): Uncertainty-aware Instance Segmentation using Dropout Sampling. Doug Morrison, Anton Milan, Epameinondas Antonakos.
- 11:30 - 12:00 - Invited Talk: Andreas Geiger (University of Tübingen). Beyond Detection: Towards Multi-Object Tracking and Segmentation.
- 12:00 - 14:00 - Lunch Break
- 14:00 - 14:30 - Invited Talk: Jana Kosecka (George Mason University) “Self-supervised and weakly supervised object detection.”
- 14:30 - 15:00 - Invited Talk: Andrew Gordon Wilson (Cornell University) Uncertainty, Loss Valleys, and Generalization in Deep Learning
- 15:00 - 15:15 - Invited Talk: Steven Waslander (University of Toronto) BayesOD: Bayesian inference for fusing epistemic and aleatoric uncertainty in deep object detectors
- 15:15 - 16:00 - Coffee Break
- 16:00 - 16:15 - Outlook and Future Challenges
- 16:15 - 16:30 - Discussion and Closing Remarks
Results of the Probabilistic Object Detection Competition
Place | Team | Paper |
---|---|---|
1st | Chuan-Wei Wang, Chin-An Cheng, Ching-Ju Cheng, Hou-Ning Hu, Hung-Kuo Chu, Min Sun. National Tsing Hua University. | AugPOD: Augmentation-oriented Probabilistic Object Detection |
2nd | Phil Ammirato, Alexander C. Berg. UNC Chapel Hill. | A Mask-RCNN Baseline for Probabilistic Object Detection |
3rd | Dongxu Li, Chenchen Xu, Yang Liu, Zhenyue Qin. The Australian National University (ANU). | Probabilistic Object Detection via Staged Non-Suppression Ensembling |
4th | Doug Morrison, Anton Milan, Epameinondas Antonakos. Queensland University of Technology (QUT) & Amazon. | Uncertainty-aware Instance Segmentation using Dropout Sampling |
Detailed results of the top four participating teams, as evaluated by the PDQ measure:
Place | PDQ Score | Average Overall Quality | Average Spatial Quality | Average Label Quality | True Positives | False Positives | False Negatives |
---|---|---|---|---|---|---|---|
1st | 22.563 | 0.605 | 0.454 | 1.000 | 152967.0 | 113620.0 | 143400.0 |
2nd | 21.432 | 0.662 | 0.503 | 0.999 | 125332.0 | 90853.0 | 171035.0 |
3rd | 20.019 | 0.655 | 0.497 | 1.000 | 118678.0 | 91735.0 | 177689.0 |
4th | 14.650 | 0.578 | 0.479 | 0.853 | 87438.0 | 48327.0 | 208929.0 |
Participate in the Competition
The competition server is now closed, but will re-open around September 2019 for the second round of this challenge. It will be presented at our workshop at IROS in November 2019.
To participate in the competition, and for more information around the data and submission format, please go to our Codalab page.
Our first challenge requires participants to detect objects in video data (from high-fidelity simulation). As a novelty, our evaluation metric rewards accurate estimates of spatial and semantic uncertainty using probabilistic bounding boxes. We developed a new probability-based detection quality (PDQ) evaluation measure for this challenge, please see the arxiv paper for more details.
Submissions must be accompanied by a 3-6 page paper explaining the method and external data used. Please use the CVPR paper format (no need to keep it double-blind) and upload your paper through our submission page on (CMT). Top performing submissions from the challenge will be invited to present their methods at the workshop. A total of $5000 AUD prize money will be rewarded to the competitors, subject to the rules explained on the Codalab page.
Important Dates
- 17 May 2019 Final Submissions to the Evaluation Server via Codalab
- 19 May 2019 Paper Submission via CMT
- 23 May 2019 Winner Announcements and Workshop Invitations
- 17 June 2019 Workshop at CVPR
Organisers
The Robotic Vision Challenges organisers are with the Australian Centre for Robotic Vision and Google AI.
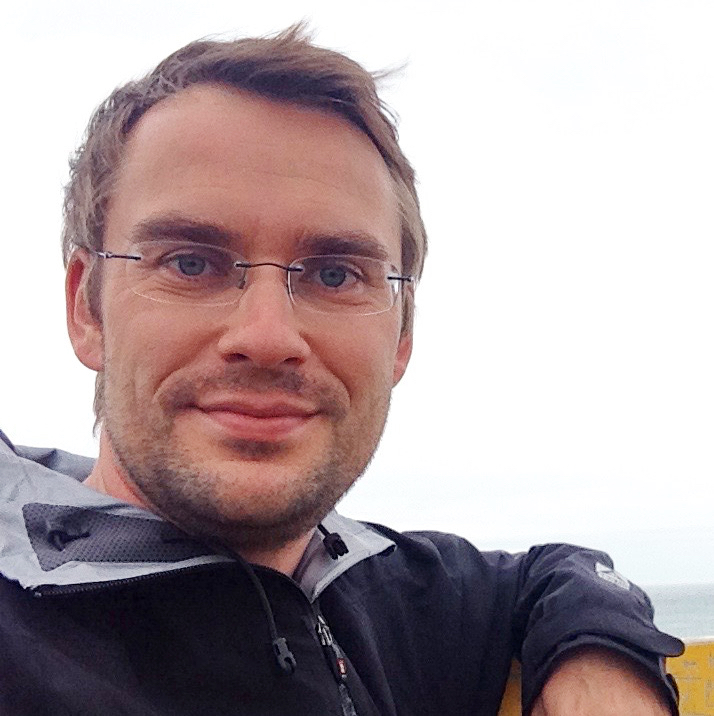
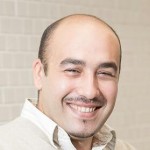
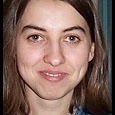
Queensland University of Technology
Queensland University of Technology
Google Brain
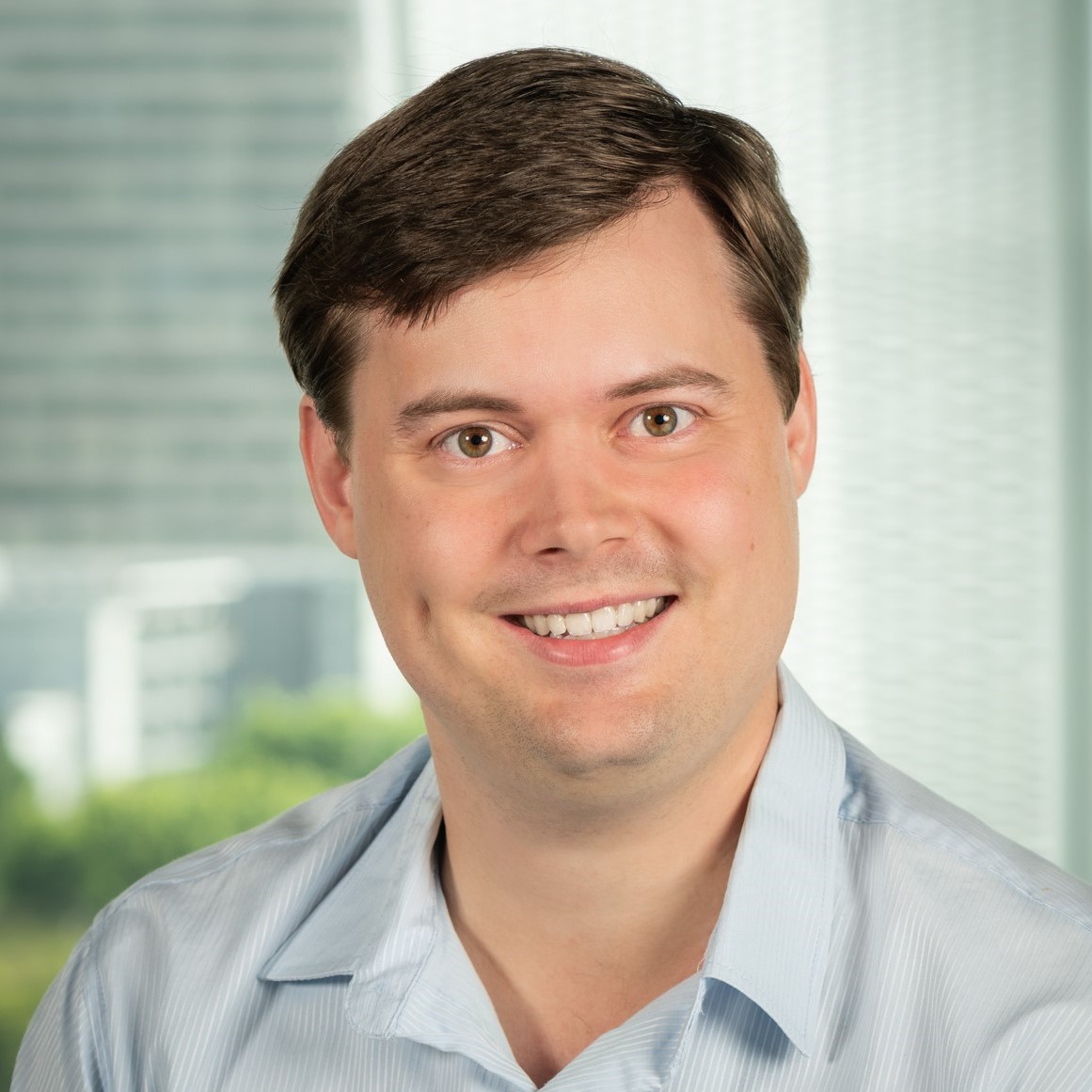
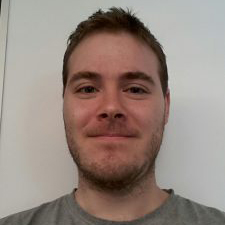
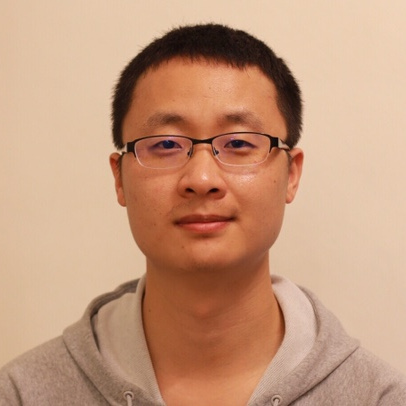
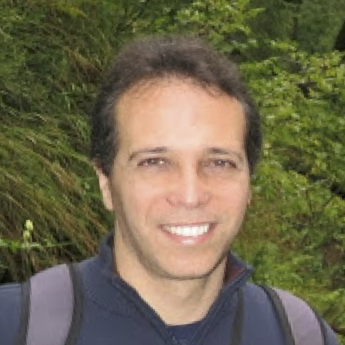
Queensland University of Technology
Queensland University of Technology
Queensland University of Technology
University of Adelaide