Introduction
Big benchmark challenges like ILSVRC or COCO supported much of the remarkable progress in computer vision and deep learning over the past years.
We aim to recreate this success for robotic vision.
We develop a set of new benchmark challenges specifically for robotic vision, and evaluate:
- scene understanding,
- uncertainty estimation,
- continuous learning for domain adaptation, and to incorporate previuosly unseen classes,
- active learning,
- active vision, and eventually
- complex tasks requiring a combination of scene understanding, learning, navigation, and interaction with the environment.
We combine the variety and complexity of real-world data with the flexibility of synthetic graphics and physics engines.
Second Challenge - Robotic Scene Understanding
The Robotic Vision Scene Understanding Challenge evaluates how well a robotic vision system can understand the semantic and geometric aspects of its environment. There are two tasks in this challenge: Object-based Semantic Mapping / SLAM, and Scene Change Detection.
We have recently concluded an iteration a CVPR2023 workshop. Please see our challenge page for further details. Details on past challenges can be found at the following links: CVPR2022, CVPR2021.
First Challenge - Probabilistic Object Detection (PrOD)
Our probabilistic object detection challenge requires participants to detect objects in video data from high-fidelity simulation. As a novelty, our evaluation metric rewards accurate estimates of spatial and semantic uncertainty using probabilistic bounding boxes. To participate and for more information around the dataset read more here.
There is no currently active PrOD challenge with prize money available but we do have a continuous evaluation server with its own test set available to promote research in the field of probabilistic object detection. The continuous evaluation server is running here.
Past official PrOD challenges can be found at the following links: ECCV2020, CVPR2019.
News
19 June 2023 CVPR 2023 Embodied AI Workshop
06 June 2023 Winning teams for RVSU 2023 Challenge announced
02 May 2023 RVSU 2023 Challenge due date extended to May 27th
15 March 2023 RVSU 2023 Challenge officially launched alongside Embodied AI Workshop! Evaluation page now up and running on EvalAI
18 January 2023 Prizes announced for 2023 challenge. 1 RTX A6000 and up to 5 Jetson Nanos to be awarded for each of the top two teams.
16 Dec 2022 Embodied AI workshop confirmed for CVPR2023 hosting the latest Scene Understanding Challenge. Workshop date - June 19th 2023.
31 May 2022 Extended deadline of CVPR 2022 Scene Understanding Challenge to June 7th
14 February 2022 We launched the second iterations of the Scene Understanding Challenge in association with the CVPR2021 Embodied AI workshop.
17 May 2021 Extended deadline of CVPR 2021 Scene Understanding Challenge to May 31st
29 March 2021 Nvidia updates our GPU prizes on offer from Titan RTX to Quadro RTX6000 GPUs!
17 February 2021 We launch the second iteration of the Scene Understanding Challenge in association with the CVPR2021 Embodied AI workshop (deadline 7th May 2021). $,2500 USD in cash and GPU prizes are available to the best teams.
29 June 2020 Nvidia kindly supports the Scene Understanding Challenge by providing 2 GPUs as prizes for the best teams. Thank you Nvidia!
8 June 2020: We released the Scene Understanding Challenge and opened it for participation (deadline 1 September 2020). $,2500 USD in cash prizes are available to the best teams.
January 2020 Our workshop proposal on the topic of Scene Understanding and Semantic SLAM: Progress, Applications, and Limitations for ICRA 2020 in Paris, France was accepted.
November 2019 We organised a workshop at IROS 2019 on the The Importance of Uncertainty in Deep Learning for Robotics. There we presented our second challenge, the Robotic Vision Scene Understanding challenge for the first time.
September 2019 Two papers got accepted for publication: Probabilistic Object Detection: Definition and Evaluation will appear in the IEEE Winter Conference on Applications of Computer Vision (WACV) in March 2020. The Nature Machine Intelligence journal published our article A probabilistic challenge for object detection in its September issue.
August 2019 We opened our evaluation servers again for the 2nd Probabilistic Object Detection Challenge. Submissions are welcome until 8 October, and the results will be presented at our workshop at IROS in November.
May 2019: We will organise a workshop at IROS 2019 on the topic of The Importance of Uncertainty in Deep Learning for Robotics. Stay tuned for further details.
January 2019: We are happy to announce that CVPR 2019 is hosting our workshop. Participants of our Robotic Vision object detection challenge will present their approaches and results, and we will announce the competition winners at the workshop.
December 2018: We released our first Robotic Vision object detection challenge, requiring object detection on video data and rewarding accurate estimates of spatial and semantic uncertainty.
June 2018: We presented our initial ideas for new benchmarks and metrics at two workshops during CVPR and RSS. Thanks to all who engaged in discussions and shared their thoughts during the workshops on Real-World Challenges and New Benchmarks for Deep Learning in Robotic Vision at CVPR, and New Benchmarks, Metrics, and Competitions for Robotic Learning at RSS.
Stay in touch and follow us on Twitter for news and announcements: @robVisChallenge.
Motivation
Big computer vision challenges and competitions like ILSVRC or COCO had a significant influence on the advancements in object recognition, object detection, semantic segmentation, image captioning, and visual question answering in recent years. These challenges posed motivating problems to the research community and proposed datasets and evaluation metrics that allowed to compare different approaches in a standardized way.
However, visual perception for robotics faces challenges that are not well covered or evaluated by the existing benchmarks. These challenges comprise calibrated uncertainty estimation, continuous learning for domain adaptation and incorporation of novel classes, active learning, and active vision.
There is currently a lack of meaningful standardised evaluation protocols and benchmarks for these research challenges. This is a significant roadblock for the evolution of robotic vision, and impedes reproducible and comparable research.
We believe that by posing a new robotic vision challenge to the research community, we can motivate computer vision and robotic vision researchers around the world to develop solutions that lead to more capable, more robust, and more widely applicable robotic vision systems.
Organisers, Support, and Acknowledgements
Stay in touch and follow us on Twitter for news and announcements: @robVisChallenge.
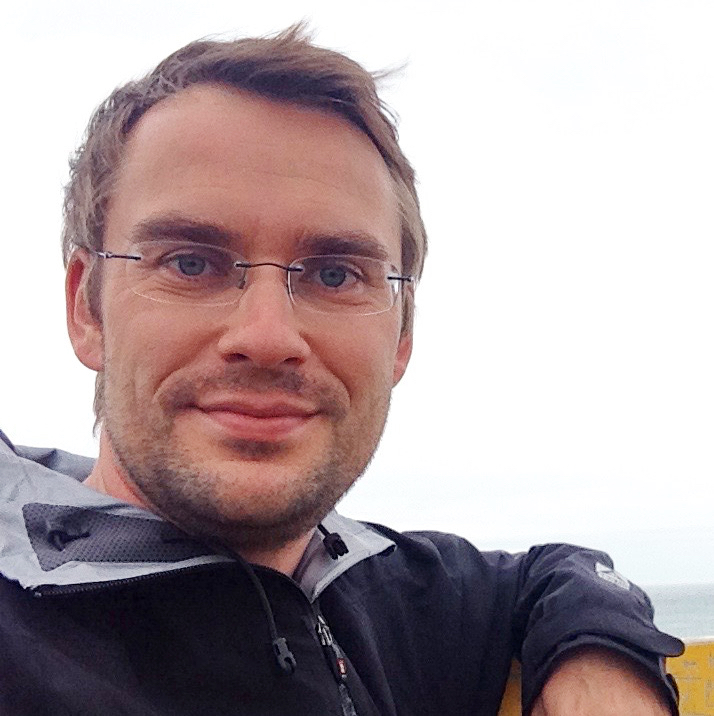
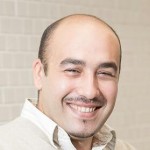
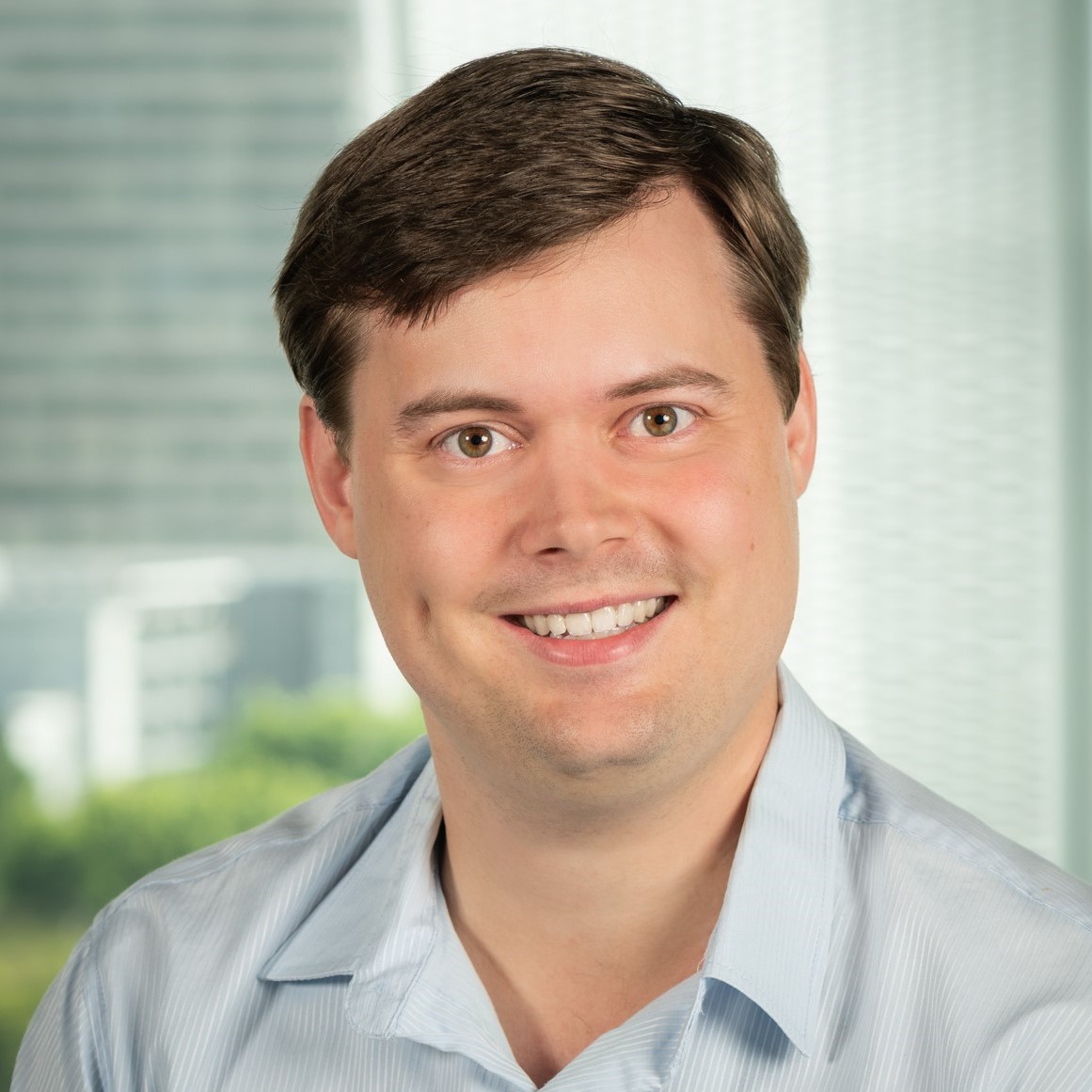
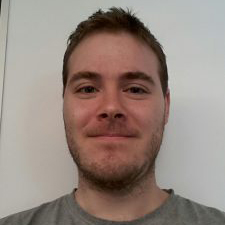
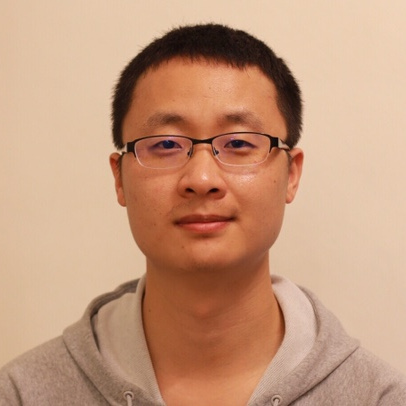
Commonwealth Scientific and Industrial Research Organisation
(formerly Queensland University of Technology)
current contributor
Queensland University of Technology
past contributor
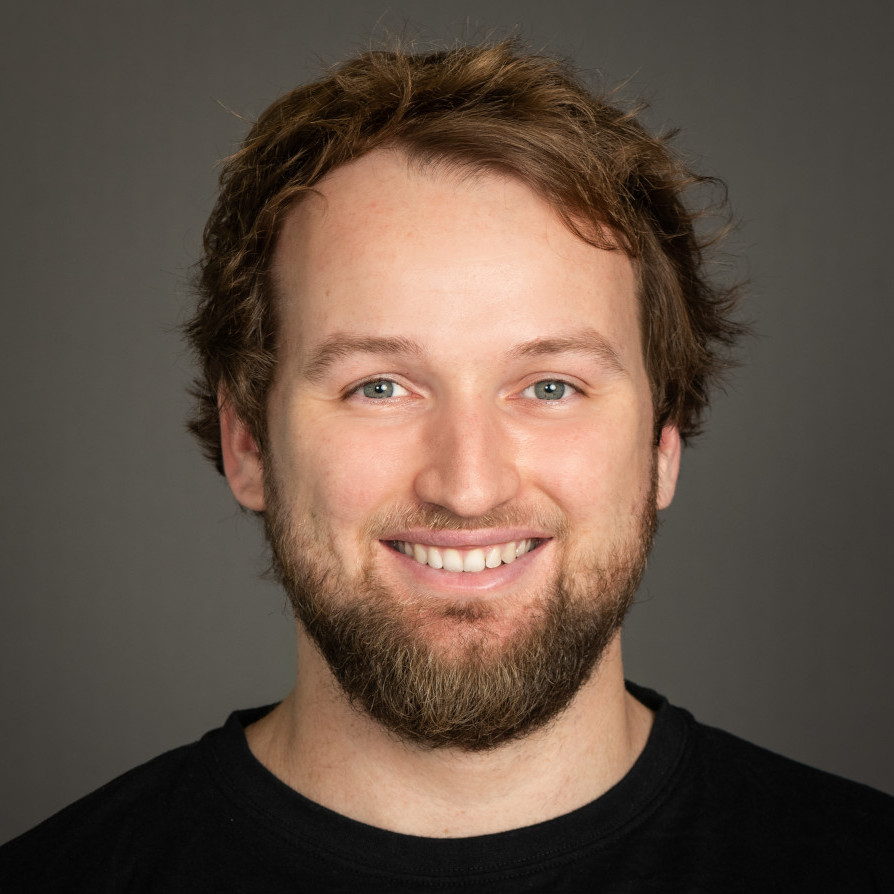
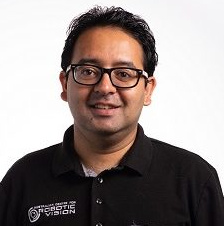
Queensland University of Technology
past contributor
The Robotic Vision Challenges organisers have been associated with the Australian Centre for Robotic Vision and QUT Centre for Robotics at Queensland University of Technology (QUT) in Brisbane, Australia. In 2023 David Hall has continued work on the challenges, supported by the Commonwealth Scientific and Industrial Research Organisation (CSIRO).
This project was supported by a Google Faculty Research Award to Niko Sünderhauf in 2018. Further support is currently provided by Nvidia.
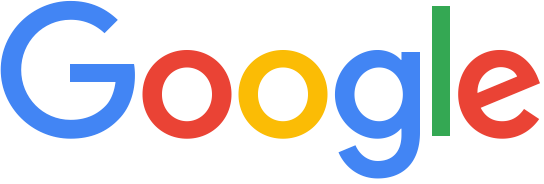
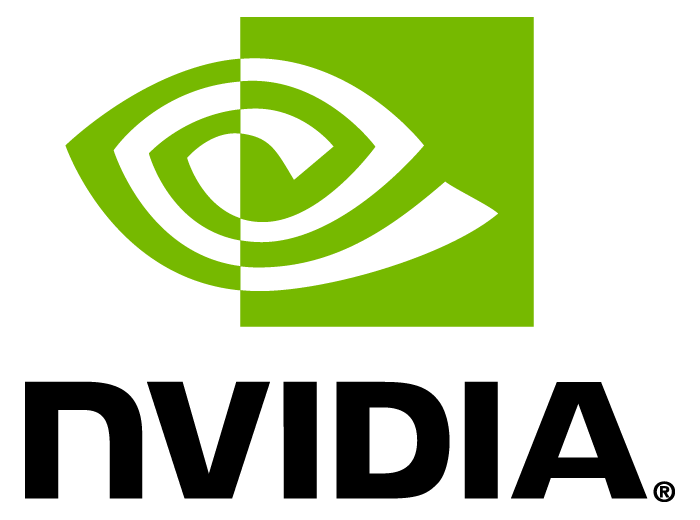
Supporters
We thank the following supporters for their valuable input and engaging discussions.
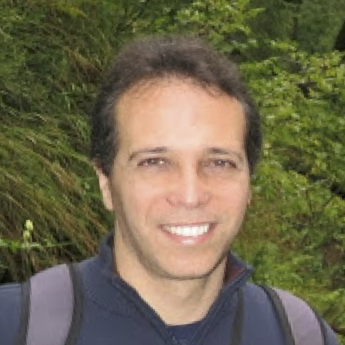
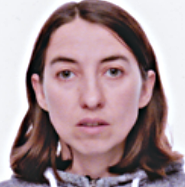
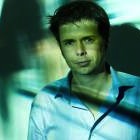
University of Adelaide
Google Brain
University of Adelaide