Our Research
We work on novel approaches to SLAM (Simultaneous Localization and Mapping) that create semantically meaningful maps by combining geometric and semantic information.
We believe such semantically enriched maps will help robots understand our complex world and will ultimately increase the range and sophistication of interactions that robots can have in domestic and industrial deployment scenarios.
In our research, we tightly combine modern deep learning and computer vision approaches with classical probabilistic robotics.
News
September 2018 Natalie Jablonsky’s paper (under review) investigates how prior knowledge about the expected scene geometry can help improve object-oriented SLAM and implements a semantically informed global orientation factor for QuadricSLAM.
August 2018: Our paper on QuadricSLAM got accepted at the IEEE Robotics and Automation Letters Journal (RA-L).
June 2018: We presented our work on QuadricSLAM at the workshop on Deep Learning for Visual SLAM in conjunction with CVPR in Salt Lake City.
May 2018: Best workshop paper award for our paper QuadricSLAM: Constrained Dual Quadrics from Object Detections as Landmarks in Semantic SLAM at the workshop on Representing a Complex World, in conjunction with the IEEE International Conference on Robotics and Automation (ICRA) in Brisbane!
Team
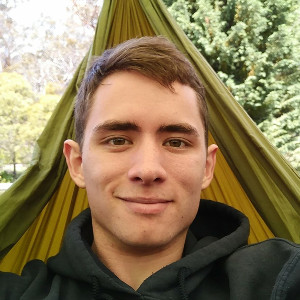
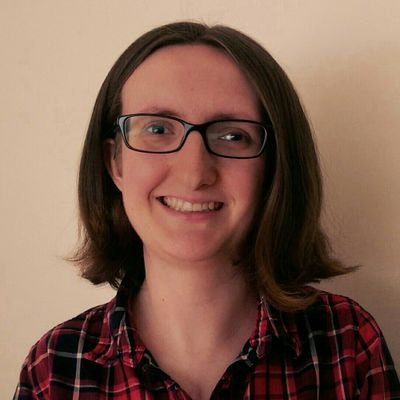
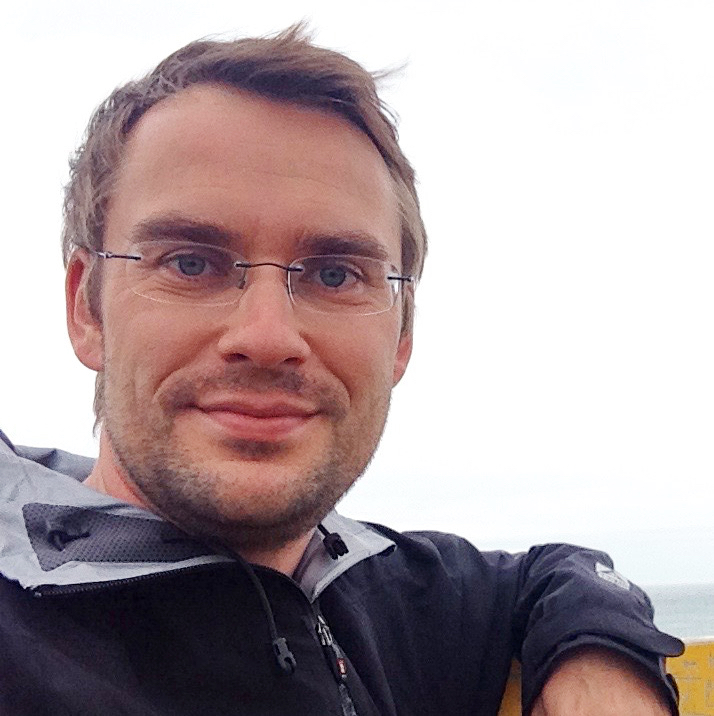
PhD Student
Queensland University of Technology
PhD Student
Queensland University of Technology
Supporters
Our team of researchers is part of the Australian Centre for Robotic Vision and based at Queensland University of Technology in Brisbane, Australia.
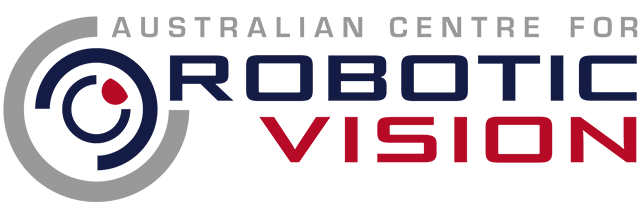
We thank the following supporters for their valuable input and engaging discussions, as well as their help in earlier research and publications.
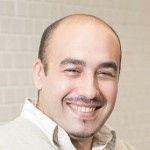
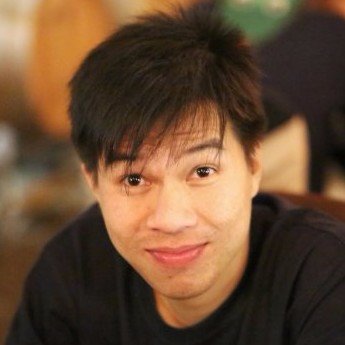
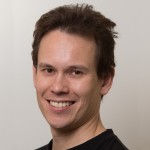